Research
We are a computational materials science research group based in the Department of Materials Science and Engineering at MIT. Our work is focused on elucidating the mechanisms of microstructural evolution for systems of relevance in materials science broadly construed. We employ a combination of computational, theoretical, and data-driven techniques to perform physics-based modeling of materials.
Check the latest poster summarizing our research focus here.
Crystallization and solid-liquid interfaces
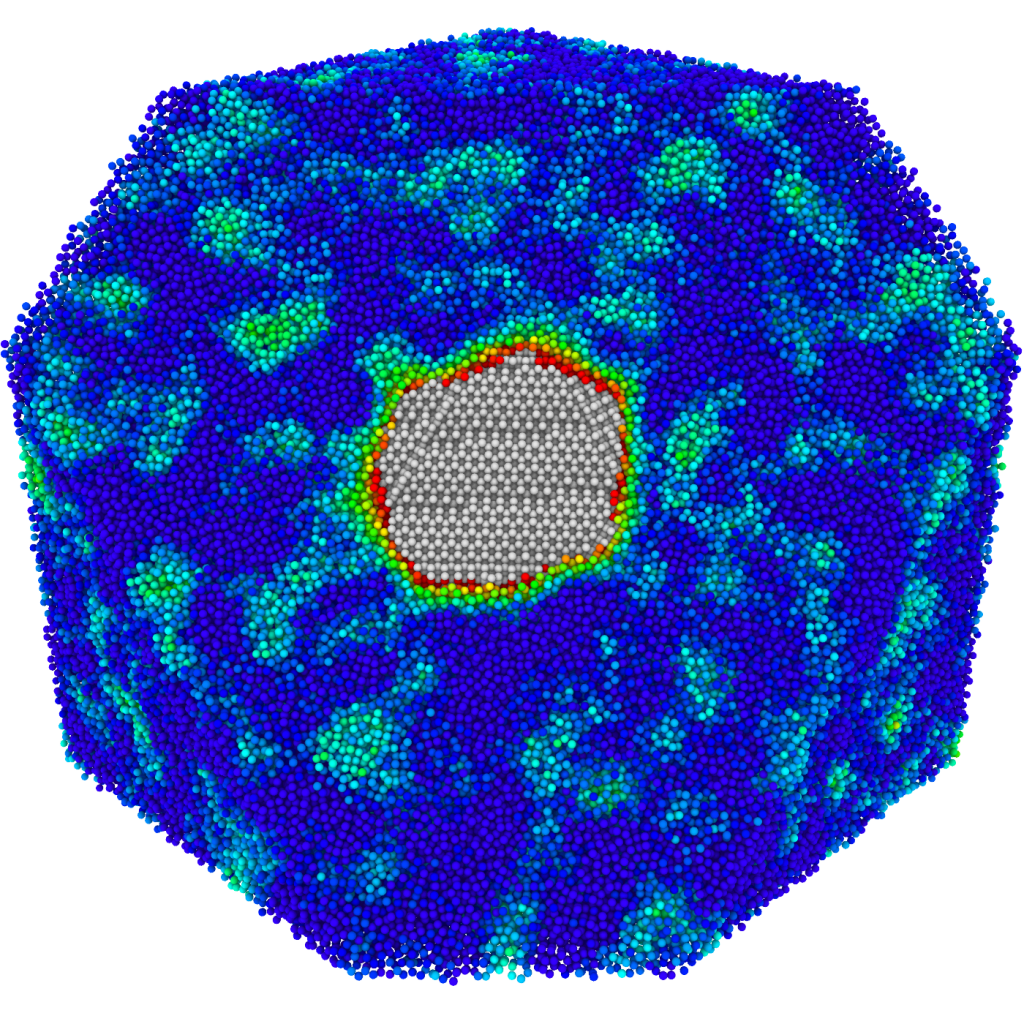
Solidification from the melt is a pervasive process in industry, from metal casting for structural applications to the Czochralski process for semiconductor wafer growth for electronics. It is important to control and understand the crystal growth process because it is at this stage that the material’s microstructure morphology is formed, which in turn defines the material’s mechanical, transport, and thermal properties.
We aim to develop models of crystal growth that are predictive and account for the nonequilibrium and multiscale behavior of materials during solidification. Our approach is derived from atomistics principles and contains a physics-based description microstructural elements.
Microstructural evolution
Atomic-level modeling performed at large scales enables the investigation of mesoscale materials properties while taking into account the contribution of each constituent atom individually. The spatial complexity of such cross-scale simulations renders them unsuitable for simple human visual inspection and challenging to post-process, severely limiting the amount and quality of scientific information that can be extracted.
Our work in this area involves the development of specialized structure characterization techniques to aid interpretation of cross-scale simulations. We aim to better understand the interplay between thermodynamic and kinetic forces that govern the process of microstructure evolution.
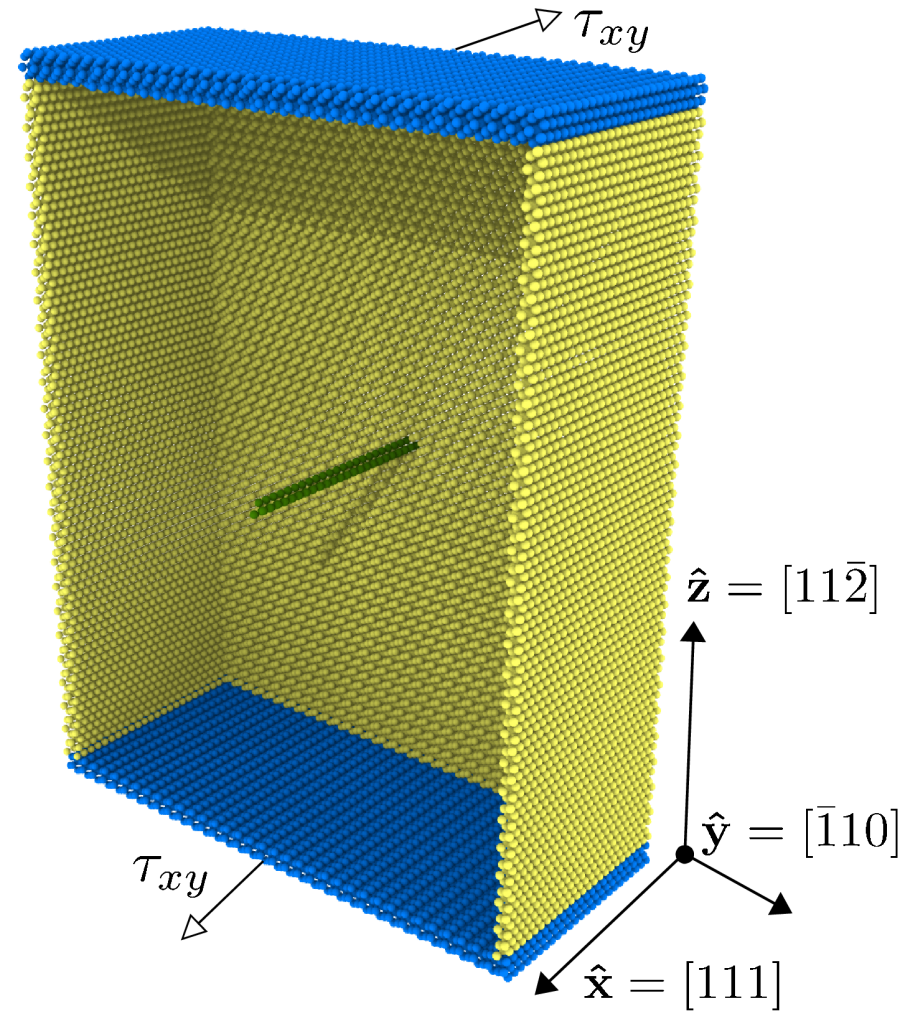
Physics-based materials modeling with Machine Learning
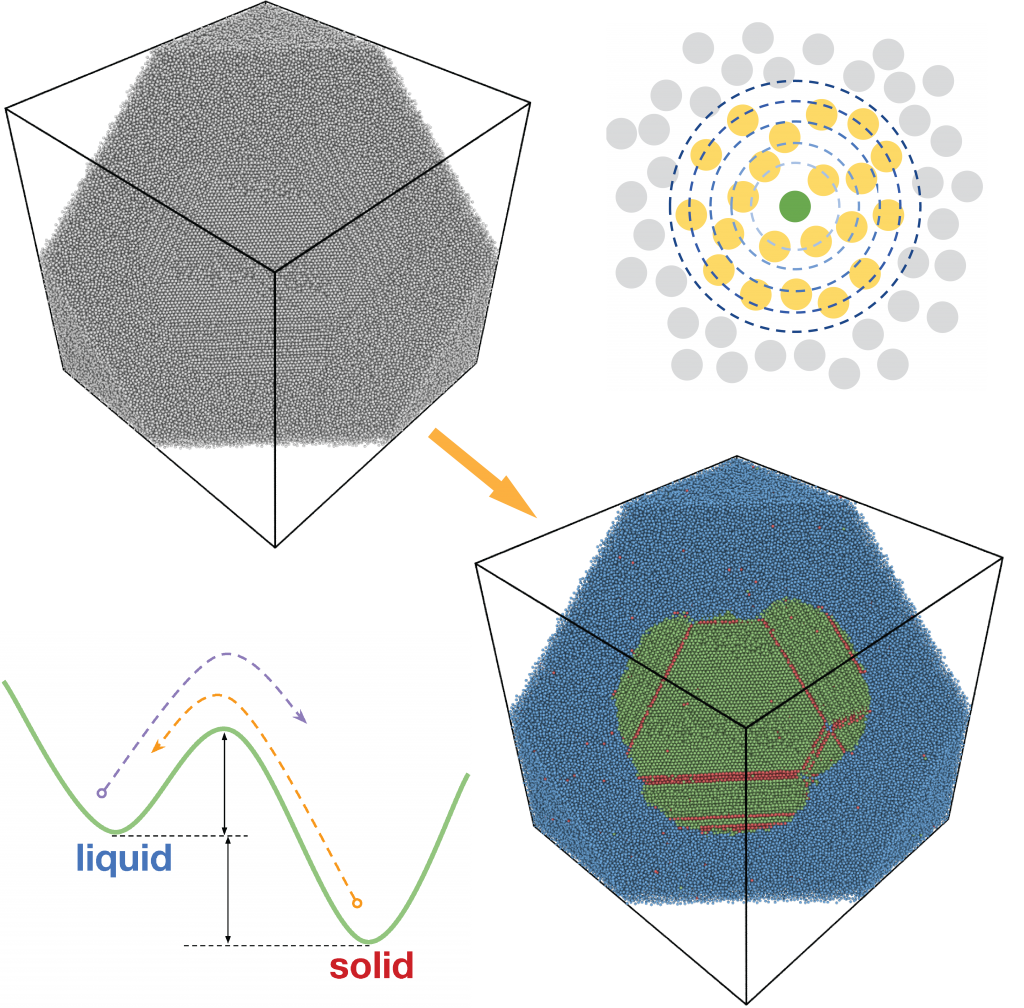
We perform physics-based modeling of materials by blending prevailing scientific methods with Machine Learning frameworks, producing physically-consistent models and conceptual knowledge. By leveraging Machine Learning as an aid to augment human intuition we facilitate the computation of quantities of practical importance in materials science – such as activation energies, kinetic coefficients, free energies, and growth rates.
The algorithms developed in our research group aim to analyze atomistic simulations with unprecedented sophistication. Our goal is to disentangle the contribution of each atomistic kinetic event leading to microstructural evolution in order to uncover the emergence of structure-property relationships.